Deep Learning Model Matches Radiologists in Detecting Significant Prostate Cancer on MRI: Study
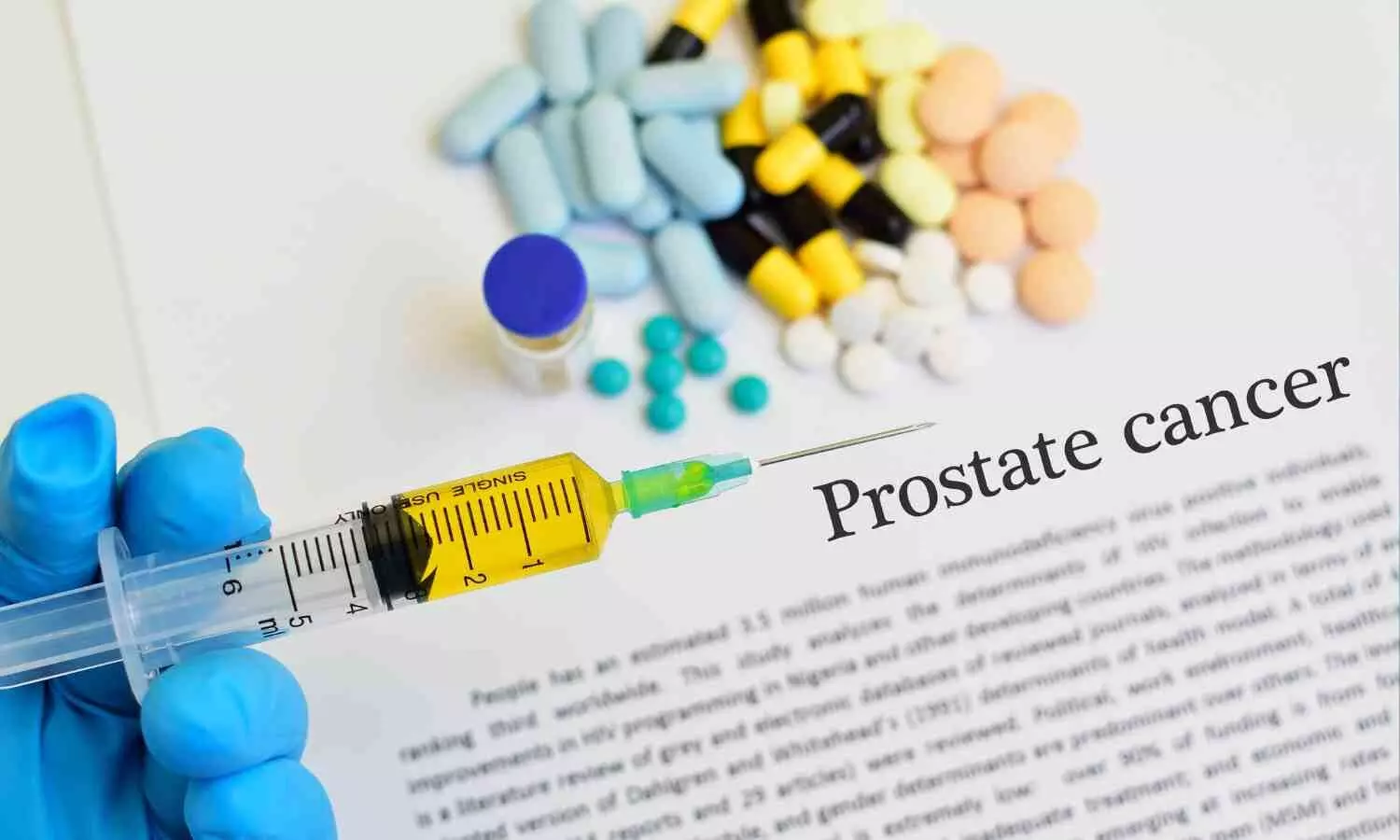
USA: A retrospective study suggested that a deep learning model matched the performance of radiologists in detecting clinically significant prostate cancer using multiparametric MRI. The findings were published online in Radiology Journal.
In an internal test set of 400 exams, the researchers found that the deep learning model demonstrated performance comparable to experienced radiologists in detecting clinically significant prostate cancer, with both achieving an area under the receiver operating characteristic curve (AUC) of 0.89.
Prostate cancer is cancer
that occurs in the prostate. The prostate is a small walnut-shaped gland in
males that produces the seminal fluid that nourishes and transports sperm. Prostate
cancer is one of the most common types of cancer. Multiparametric MRI of the prostate is a standard imaging
method used to diagnose clinically significant prostate cancer (csPCa) and
assist in targeting lesions during a biopsy. Considering this Jason C. Cai, et
al conducted a study to create a deep learning model using patient-level labels
to predict the presence of clinically significant prostate cancer (csPCa)
without information on tumor location and to compare its performance with that
of radiologists.
To achieve this, the
research team included 5,735 patients in the study, of whom 5,215 were male.
Out of these, 1,514 examinations (involving 1,454 patients) revealed clinically
significant prostate cancer (csPCa) and were conducted between January 2017 and
December 2019. For the internal test set, radiology reports were utilized,
while PI-RADS ratings were used for the external ProstateX test set. The study
compared performance using areas under the receiver operating characteristic
curves (AUCs) and the DeLong test and employed gradient-weighted class
activation maps (Grad-CAMs) to illustrate tumor localization.
They found the following
results: –
·
In the internal test set, which included 400
examinations, both the deep learning (DL) classifier and radiologists achieved
an AUC of 0.89. In the external test set, consisting of 204 examinations, the
DL classifier had an AUC of 0.86, while radiologists had an AUC of 0.84.
·
The combination of the DL classifier
and radiologists achieved an AUC of 0.89.
·
Grad-CAMs showed activation over the csPCa
lesions in 35 out of 38 true-positive cases in the internal test set and in 56
out of 58 true-positive cases in the external test set.
“The
deep learning model is expected to enhance the detection of prostate cancer and
improve the diagnostic accuracy of radiologists”, the researchers concluded.
Reference
Cai,
J. C., Nakai, H., Kuanar, S., Froemming, A. T., Bolan, C. W., Kawashima, A.,
Takahashi, H., Mynderse, L. A., Dora, C. D., Humphreys, M. R., Korfiatis, P.,
Rouzrokh, P., Bratt, A. K., Conte, G. M., Erickson, B. J., & Takahashi, N.
(2024). Fully Automated Deep Learning Model to Detect Clinically Significant
Prostate Cancer at MRI. Radiology, 312(2), e232635.
https://doi.org/10.1148/radiol.232635